Human-in-the-Loop: How Human Oversight is Shaping the Future of Machine Learning
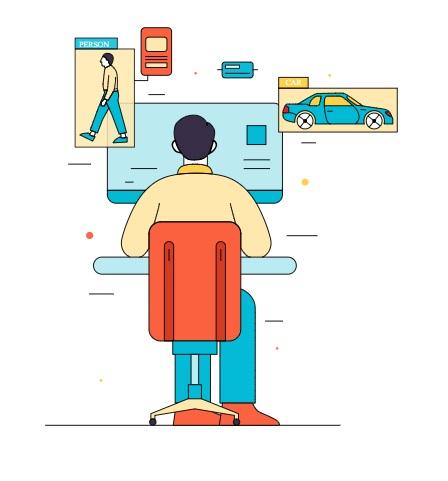
Introduction to Human-in-the-Loop (HITL)
Welcome to the realm where humans and machines collaborate harmoniously – Human-in-the-Loop (HITL). Imagine a world where human oversight fuels the advancement of machine learning, shaping the future of technology as we know it. In this blog post, we delve into the pivotal role that human intervention plays in refining algorithms, enhancing AI capabilities, and ultimately revolutionizing industries across the globe. Join us on a journey through the exciting landscape of HITL and discover how this synergy is transforming our digital landscape.
The Role of Human Oversight in Machine Learning
Machine learning algorithms have revolutionized the way we process data and make decisions. However, they are not infallible. Human oversight is essential in ensuring that these algorithms perform accurately and ethically. One critical role humans play in machine learning is providing labeled data for training models. This labeling process helps algorithms learn patterns and make predictions effectively.
Moreover, human intervention is crucial in monitoring algorithm performance and making adjustments when needed. Humans can identify biases or errors in the system that machines may overlook. This oversight ensures fair outcomes and prevents potentially harmful decisions from being made based on flawed data.
Collaboration between humans and machines creates a checks-and-balances system that optimizes the capabilities of both parties. The synergy between human expertise and machine efficiency results in more reliable AI systems that benefit society as a whole.
Advantages of Human-in-the-Loop
Human-in-the-Loop (HITL) brings a unique blend of human intelligence and machine efficiency to the table. One key advantage is the ability to improve algorithm accuracy by incorporating human feedback. This iterative process allows for continuous learning and adaptation, leading to more precise outcomes.
Moreover, HITL enables handling complex tasks that machines alone may struggle with, such as nuanced language understanding or image recognition in ambiguous scenarios. The human oversight ensures a level of contextual comprehension that enhances decision-making processes within machine learning systems.
Additionally, HITL promotes transparency and accountability in AI applications. By having humans involved in the loop, it helps prevent biases from being perpetuated or unintended consequences from occurring. This collaborative approach fosters trust between users and AI technologies while also fostering innovation through diverse perspectives.
In essence, Human-in-the-Loop serves as a bridge between the capabilities of artificial intelligence and the cognitive abilities of humans, creating synergies that drive advancements across various industries.
Challenges and Limitations of Human-in-the-Loop
Human-in-the-Loop (HITL) may offer a powerful solution to the limitations of fully automated machine learning systems. However, it also comes with its own set of challenges and constraints that must be carefully considered.
One major challenge is the potential for bias to be introduced through human oversight. Humans are susceptible to their own biases, which can inadvertently influence the data labeling process and ultimately impact the performance of the machine learning model.
Additionally, scalability can be an issue with HITL as it requires continuous human intervention, making it more resource-intensive compared to fully autonomous systems. This could pose difficulties in large-scale applications where rapid decision-making is crucial.
Furthermore, ensuring consistent quality control and maintaining a high level of accuracy in human-labeled data sets can be another obstacle. Human errors or inconsistencies in labeling tasks can lead to suboptimal performance in machine learning algorithms.
Despite these challenges, organizations are exploring ways to mitigate these limitations by implementing robust processes for training and monitoring human annotators. The ongoing development of tools and technologies aimed at streamlining the human-machine collaboration process is key to overcoming these obstacles as we continue shaping the future of machine learning with HITL.
Real-life Examples of Human-in-the-Loop Applications
In the realm of healthcare, Human-in-the-Loop technology is revolutionizing medical imaging analysis. Radiologists can use AI algorithms to detect anomalies in scans, while human oversight ensures accurate diagnosis and treatment planning.
In e-commerce, HITL is enhancing product recommendations by combining machine learning algorithms with human feedback. This hybrid approach helps tailor suggestions based on customer preferences and behavior patterns.
HITL is also making waves in content moderation on social media platforms. By incorporating human reviewers into automated systems, inappropriate content can be swiftly identified and removed from users' feeds.
Moreover, autonomous vehicles are benefiting from HITL applications where AI-driven sensors analyze road conditions, with humans stepping in to make critical decisions in complex traffic scenarios.
Real-life Examples of HITL Applications showcase the powerful synergy between artificial intelligence and human expertise across various industries.
Criticisms and Controversies Surrounding HITL
Criticisms and controversies surrounding Human-in-the-Loop (HITL) have sparked debates in the tech world. Some argue that reliance on human oversight can be a bottleneck, slowing down the machine learning process. Skeptics raise concerns about potential biases introduced by humans, impacting the accuracy of algorithms.
Others question the scalability of HITL systems, especially as data volumes continue to grow exponentially. The need for continuous human involvement in training data sets is seen as a potential hindrance to efficiency and automation in machine learning models.
Additionally, there are ethical dilemmas surrounding HITL, such as privacy issues and consent when using personal data for model improvement. Balancing innovation with user protection remains a challenge in this evolving landscape.
Despite these criticisms, proponents believe that HITL plays a crucial role in ensuring AI systems' accountability and transparency. Striking a balance between human judgment and automated processes is key to harnessing the full potential of machine learning technologies while addressing these valid concerns.
The Future of HITL and Its Impact on Society
As we look ahead to the future of Human-in-the-Loop (HITL) technology, one thing is certain: its impact on society will be profound. With the continued integration of human oversight in machine learning processes, we can expect greater transparency and accountability in AI systems. This means that decisions made by algorithms will be more explainable and understandable to users.
Moreover, HITL has the potential to enhance not only technological advancements but also ethical considerations in AI development. By involving humans in the loop, we can ensure that biases are mitigated and that AI systems reflect diverse perspectives.
In terms of practical implications, HITL could revolutionize industries such as healthcare, finance, and transportation by improving accuracy and reliability in decision-making processes. However, challenges around data privacy and security must be carefully navigated to fully realize the benefits of this approach.
The future of HITL holds promise for a more responsible and inclusive use of artificial intelligence technologies across various sectors by outsourcing to data labeling services.
Conclusion
Human-in-the-Loop (HITL) represents a pivotal approach that combines the power of machines with human intelligence to enhance the capabilities of artificial intelligence systems. The synergy between humans and machines in HITL is reshaping the landscape of machine learning by ensuring accuracy, reliability, and ethical decision-making. As we navigate through the challenges and opportunities presented by HITL, it is evident that human oversight will continue to play a crucial role in shaping the future of AI technologies. Embracing this collaborative model can lead us towards a more responsible and sustainable development of intelligent systems that benefit society as a whole.
- Art
- Causes
- Crafts
- Dance
- Drinks
- Film
- Fitness
- Food
- Игры
- Gardening
- Health
- Главная
- Literature
- Music
- Networking
- Другое
- Party
- Religion
- Shopping
- Sports
- Theater
- Wellness
- IT, Cloud, Software and Technology