Leveraging Text Analytics for Customer Feedback Analysis
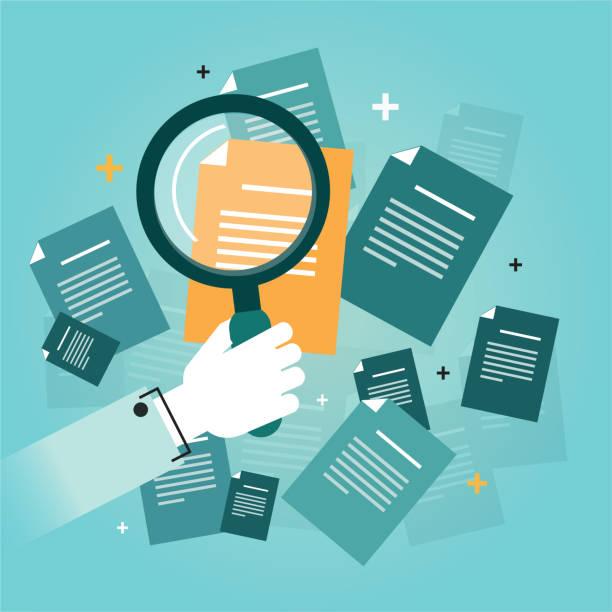
Unstructured text makes up an important part of the massive volumes of data created every second in the digital age. Finding patterns and important insights in textual data is the practice of text analytics, a branch of data analytics. Through the use of computational approaches, unstructured text is transformed into a structured format, enabling companies to base data-driven choices on qualitative information.
1. Text Analytics: What Is It?
Text analytics is the analysis of textual data using a combination of statistical techniques, machine learning, and natural language processing (NLP). The objective is to extract meaningful data from text, such as relationships, subjects, feelings, and trends, so that strategic planning and decision-making may be done. Numerous text sources, including as emails, research papers, social network postings, and consumer reviews, may be processed using this method.
2. Important Text Analytics Techniques
Text analytics is based on many key techniques:
a.Finding patterns, trends, and connections in big unstructured text databases is known as text mining. Text mining can reveal previously undiscovered information, such as commonly discussed subjects or new patterns in consumer reviews.
b.Sentiment analysis is a technique used to evaluate a text’s sentiment or emotional tone. Sentiment analysis is a widely used technique in customer feedback analysis and social media monitoring to ascertain if the overall sentiment is neutral, positive, or negative.
c.The process of topic modeling involves determining the primary subjects or ideas present in a group of texts. This is especially helpful for comprehending the overarching themes found in big datasets, such research articles or consumer evaluations.
d.Named Entity Recognition (NER): NER recognizes and categorizes important textual entities, including names of individuals, groups, places, and dates. This facilitates the organization and extraction of pertinent data from huge databases.
e. Text classification is the process of grouping text into pre-established groups. Emails, for instance, might be labeled as spam or not, and customer reviews can be divided into many categories of problems.
3. Text Analytics Applications
There are several uses for text analytics in a variety of industries:
a.Customer experience management: To enhance their offerings, businesses employ text analytics to examine consumer input from social media, reviews, and surveys. Businesses may resolve issues and raise customer satisfaction by knowing their customers’ feelings.
b.Healthcare: To enhance patient care and spot patterns in medical research, text analytics may be utilized in the analysis of clinical notes, patient testimonials, and research publications.
c.Market Research: By looking at internet reviews, social media, and industry studies, businesses employ text analytics to study market trends, customer behavior, and rival strategy.
d.Risk management: To identify possible dangers and stop fraud, financial institutions utilize text analytics to keep an eye on news, social media, and other sources.
In summary
With the help of text analytics, businesses may fully utilize the potential of unstructured data. Businesses and organizations may make better choices, increase customer happiness, and obtain a competitive advantage in their respective sectors by turning text into actionable information. With the amount of textual data increasing,Text analytics is going to become more and more important, and current data-driven tactics will not be complete without it.
- Art
- Causes
- Crafts
- Dance
- Drinks
- Film
- Fitness
- Food
- Oyunlar
- Gardening
- Health
- Home
- Literature
- Music
- Networking
- Other
- Party
- Religion
- Shopping
- Sports
- Theater
- Wellness
- IT, Cloud, Software and Technology