LLM Vulnerabilities Unveiled: The Top Five Risks of 2024
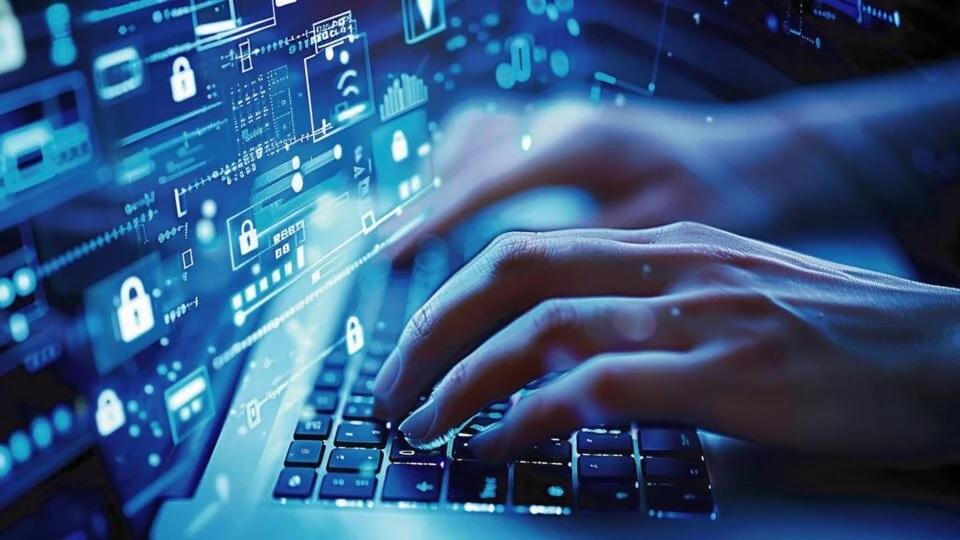
In a turn of events worthy of a sci-fi thriller, Large Language Models (LLMs) have surged in popularity over the past few years, demonstrating the adaptability of a seasoned performer and the intellectual depth of a subject matter expert.
These advanced AI models, powered by immense datasets and cutting-edge algorithms, have transformed basic queries into engaging narratives and mundane reports into compelling insights. Their impact is so significant that, according to a recent McKinsey survey, nearly 65% of organizations now utilize AI in at least one business function, with LLMs playing a pivotal role in this wave of adoption.
But are LLMs truly infallible? This question arose in June when we highlighted in a blog post how LLMs failed at seemingly simple tasks, such as counting the occurrences of a specific letter in a word like strawberry.
Backdoor Attacks
Backdoor attacks involve the deliberate insertion of subtle manipulations during the model’s training phase, designed to alter its behavior under specific conditions.
Dormant Triggers: These malicious modifications remain inactive until activated by specific inputs, making them difficult to detect.
Biased Outcomes: An attacker might inject biased data into the training set, causing the model to favor particular agendas or generate misleading outputs in certain scenarios.
Model Denial of Service (DoS)
DoS attacks target the availability of LLMs by overwhelming them with excessive requests or exploiting vulnerabilities that lead to system failure.
Input Overload: Continuous overflow or variable-length input flooding can degrade the model’s performance, disrupt service, and increase operational costs.
Developer Awareness: Many developers are unaware of these vulnerabilities, making models particularly susceptible to such attacks.
Insecure Output Handling
Failure to validate LLM outputs can expose backend systems to severe risks, such as:
Security Breaches: Vulnerabilities like cross-site scripting (XSS), cross-site request forgery (CSRF), and remote code execution may be exploited.
Data Leaks: LLMs may unintentionally reveal sensitive information, such as personally identifiable information (PII), violating privacy regulations and exposing users to identity theft.
As LLMs continue to transform industries with their capabilities, understanding and addressing their vulnerabilities is essential. While the risks are significant, disciplined practices, regular updates, and a commitment to security can ensure the benefits far outweigh the dangers.
Organizations must remain vigilant and proactive, especially in fields like cybersecurity, where the stakes are particularly high. By doing so, they can harness the full potential of LLMs while mitigating the risks posed by malicious actors.
To Know More, Read Full Article @ https://ai-techpark.com/top-2024-llm-risks/
Related Articles -
- Art
- Causes
- Crafts
- Dance
- Drinks
- Film
- Fitness
- Food
- Games
- Gardening
- Health
- Home
- Literature
- Music
- Networking
- Other
- Party
- Religion
- Shopping
- Sports
- Theater
- Wellness
- IT, Cloud, Software and Technology