Top 8 Latest Developments in Data Science Should Know About That
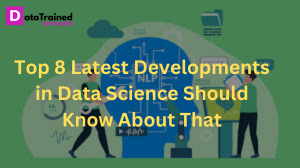
In the fast-paced world of data science, staying updated with the latest developments is crucial. With advancements happening at a rapid pace, it's essential to keep track of emerging trends and technologies. In this blog post, we'll explore 8 of the latest developments in data science that you should know about.
Advancements in Natural Language Processing (NLP):
Natural Language Processing (NLP) has seen significant advancements in recent years, thanks to breakthroughs in deep learning. Techniques like BERT (Bidirectional Encoder Representations from Transformers) and GPT-3 (Generative Pre-trained Transformer 3) have pushed the boundaries of what's possible with NLP. These models have achieved remarkable results in tasks such as sentiment analysis, text summarization, and language translation and for getting the best Data Science Course in the digital age, click here to know more about the course details, syllabus, etc
BERT, developed by Google, introduced the concept of bidirectional training for NLP tasks. It has been widely adopted and fine-tuned for various applications, leading to improvements in accuracy and performance. GPT-3, developed by OpenAI, is one of the largest language models to date, with 175 billion parameters. It has demonstrated capabilities in generating human-like text and performing a wide range of NLP tasks.
Explainable AI (XAI):
As AI models become more complex, there is a growing need for transparency and interpretability. Explainable AI (XAI) aims to address this need by providing insights into how AI models make decisions. Techniques like LIME (Local Interpretable Model-agnostic Explanations) and SHAP (SHapley Additive exPlanations) are being used to explain the predictions of black-box models.
LIME generates local approximations of complex models, allowing users to interpret individual predictions. SHAP, on the other hand, provides global explanations by assigning each feature an importance value based on its contribution to the model's output. These techniques are invaluable for understanding and trusting AI systems, especially in high-stakes domains such as healthcare and finance.
AutoML and Automated Data Science:
AutoML (Automated Machine Learning) is democratizing data science by automating the process of model selection, hyperparameter tuning, and feature engineering. Tools like Google AutoML, H2O.ai, and DataRobot are making it easier for non-experts to build and deploy machine learning models.
These platforms provide user-friendly interfaces that guide users through the entire machine learning pipeline, from data preprocessing to model evaluation. By automating repetitive tasks, AutoML allows data scientists to focus on more strategic aspects of model development, such as feature selection and model interpretation to enroll for the best data science course in Pune, best course fee guarantee with lots of payment options.
Graph Analytics:
Graph analytics is gaining traction as organizations seek to extract insights from interconnected data. Graph databases and analytics platforms are being used to model and analyze complex relationships in data, such as social networks, supply chains, and financial transactions.
Graph analytics has applications in various domains, including social network analysis, recommendation systems, and fraud detection. By representing data as a network of nodes and edges, organizations can uncover hidden patterns and relationships that traditional analytics methods might miss.
Federated Learning:
Federated learning is a decentralized approach to training machine learning models across multiple edge devices or servers. Instead of collecting data in a central repository, federated learning allows models to be trained locally on device or server while keeping data localized.
This approach has significant privacy advantages, as it reduces the need to transfer sensitive data to a central server. Federated learning has applications in healthcare, finance, and IoT, where data privacy and security are paramount.
Edge Computing and Data Science:
Edge computing is revolutionizing data science by bringing computation and data storage closer to the source of data generation. By processing data locally on edge devices or servers, organizations can reduce latency, conserve bandwidth, and enhance data privacy.
Edge computing has applications in real-time analytics, IoT, and autonomous vehicles, where low latency and high reliability are critical. By performing data processing and analytics at the edge, organizations can extract insights from data in near real-time, enabling faster decision-making and response.
Time Series Forecasting with Deep Learning:
Deep learning techniques such as Long Short-Term Memory (LSTM) and Gated Recurrent Unit (GRU) networks have revolutionized time series forecasting. These architectures are well-suited for capturing long-term dependencies in sequential data, making them ideal for time series prediction tasks.
LSTM and GRU networks have achieved state-of-the-art results in forecasting applications such as stock price prediction, weather forecasting, and energy demand forecasting. By leveraging deep learning techniques, organizations can build more accurate and robust forecasting models and if you are a resident of Delhi NCR, you can enroll now for the best Data Science Course in Delhi from DataTrained Education.
Ethical AI and Responsible Data Science:
As AI becomes more pervasive, it's essential to consider the ethical implications of data science. Responsible data science involves ensuring that AI systems are fair, transparent, and accountable. This includes addressing issues such as bias and fairness, privacy, and data governance.
By incorporating ethical considerations into the data science workflow, organizations can mitigate the risks associated with AI, build trust with users, and ensure that their systems are used responsibly. This is critical for the long-term success and adoption of AI technologies.
In conclusion, these 8 developments represent the cutting edge of data science and have the potential to transform industries and society. By staying informed about these advancements, data scientists can stay ahead of the curve and harness the full power of data to drive innovation and create value.
- data_science
- data_science_course
- data_science_courses
- data_science_training
- course_for_data_science
- top_data_science_course_provider
- best_data_scientist
- data_scientist
- python_programming
- python_training_program
- machine_learning_course_provider
- software_development_course_provider
- best_data_science_in_AI_tech
- best_AI_and_ML_courses_provider
- top_best_data_science_courses
- data_science_course_provider
- best_data_science_course_provider
- data_science_course_training
- Online_data_science_course_classes
- best_data_science_online_classes
- online_data_science_classes
- data_science_online_course
- data_science_course_classes
- data_science_course_learning
- data_science_online_classes
- data_science_online_training
- online_class_for_data_science
- data_science_courses_learning
- online_classes_in_data_science
- data_science_online_course_training
- data_science_online_courses_training
- Art
- Causes
- Crafts
- Dance
- Drinks
- Film
- Fitness
- Food
- Игры
- Gardening
- Health
- Главная
- Literature
- Music
- Networking
- Другое
- Party
- Religion
- Shopping
- Sports
- Theater
- Wellness
- IT, Cloud, Software and Technology